As businesses strive to align with current and upcoming regulations, advance towards net-zero objectives, and enhance transparency for stakeholders, many are focusing on disclosing their direct (Scope 1), indirect (Scope 2), and value chain (Scope 3) emissions. Incorporating Generative AI (GenAI) into these efforts introduces several dimensions worth considering, especially in the context of carbon footprint management. Sustainability AI delves into these aspects to guide companies in making environmentally responsible choices when deploying GenAI technologies.
Foundation and Training of Models
Foundation models, also known as large language models (LLMs), serve a wide range of applications. These models, offered by vendors pre-trained on extensive datasets, necessitate significant computational power for design and operation, which in turn demands energy. In our analysis, we distributed the emissions generated from this energy use across each corporate entity that utilised the model, promoting a shared responsibility for the emissions incurred.
Domain-Specific Model Development
The creation of domain-specific models, tailored to deliver prompt value in particular areas such as software development, taxation, or legal services, also relies on computational power and energy. The specificity of these models means they can offer more targeted solutions, but the environmental impact of their development must also be accounted for.
Customisation Practices
A best practice among businesses is to acquire licenses for both foundation and domain-specific models and then enhance them with unique data and expertise. This process of customisation not only refines the models’ effectiveness but also requires additional computational resources, further contributing to the carbon footprint.
Model Inferencing
Model inferencing, or user prompting, encompasses the routine operation of GenAI models. Whether for foundation or domain-specific applications, the day-to-day use of these technologies forms a significant part of their overall environmental impact.
Enterprise Application Integration
The integration of GenAI capabilities into enterprise applications, such as Enterprise Resource Planning (ERP) systems, introduces another layer of carbon emissions. As these applications become more AI-driven, their operational emissions footprint expands accordingly.
In our examination of the initial deployment of GenAI technologies by Sustainability AI, we considered various factors to estimate the carbon emissions associated with their use. This included assessing the labor hours, number of processors used, power consumption per processor, and an emissions factor to calculate the metric tons of carbon dioxide equivalent produced.
This comprehensive approach to evaluating the carbon footprint of GenAI applications is crucial for companies committed to reducing their environmental impact while harnessing the benefits of AI for ESG reporting and beyond. By understanding and managing the emissions associated with these technologies, businesses can make more informed decisions that align with their sustainability goals and regulatory requirements.
Awards & Accreditations
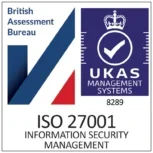
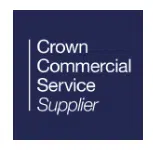
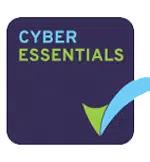
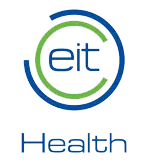
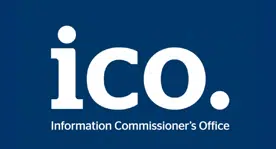
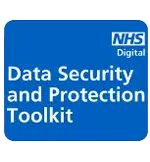